Upcoming Events
IC Spring Seminar with Guest Speaker Micah Goldblum
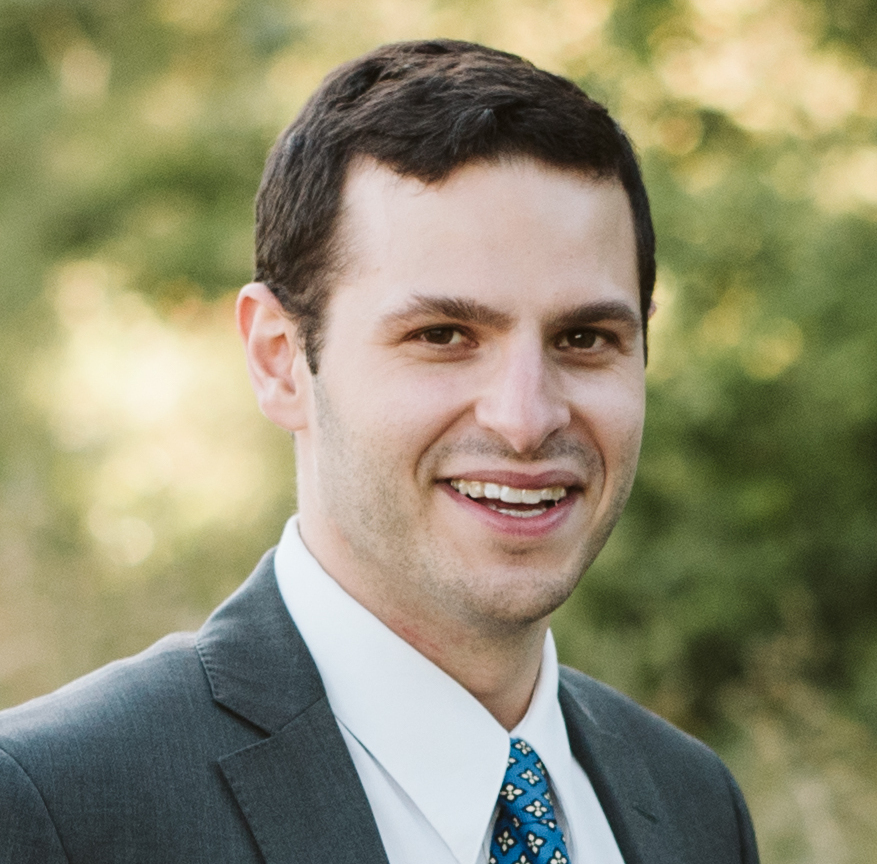
Abstract
Despite the widespread proliferation of neural networks, the mechanisms through which they operate so successfully are not well understood. In this talk, we will first explore empirical and theoretical investigations into neural network training and generalization and what they can tell us about why deep learning works. Then, we will examine a recent line of work on algorithm learning. While neural networks typically excel at pattern matching tasks, we consider whether neural networks can learn algorithms that scale to problem instances orders of magnitude larger than those seen during training.
Bio
Micah Goldblum is a postdoctoral researcher at New York University with Yann LeCun and Andrew Gordon Wilson. His research portfolio includes award winning work in Bayesian inference, generalization theory, algorithmic reasoning, and AI security and privacy. Micah’s paper on model comparison received the Outstanding Paper Award at ICML 2022. Before his current position, he received a Ph.D. in mathematics at the University of Maryland where he worked with Tom Goldstein and Wojciech Czaja.
Event Details
Media Contact
EVENTS BY SCHOOL & CENTER
School of Computational Science and Engineering
School of Interactive Computing
School of Cybersecurity and Privacy
Algorithms and Randomness Center (ARC)
Center for 21st Century Universities (C21U)
Center for Deliberate Innovation (CDI)
Center for Experimental Research in Computer Systems (CERCS)
Center for Research into Novel Computing Hierarchies (CRNCH)
Constellations Center for Equity in Computing
Institute for People and Technology (IPAT)
Institute for Robotics and Intelligent Machines (IRIM)