Upcoming Events
CSE Faculty Candidate Seminar - Meenakshi Khosla
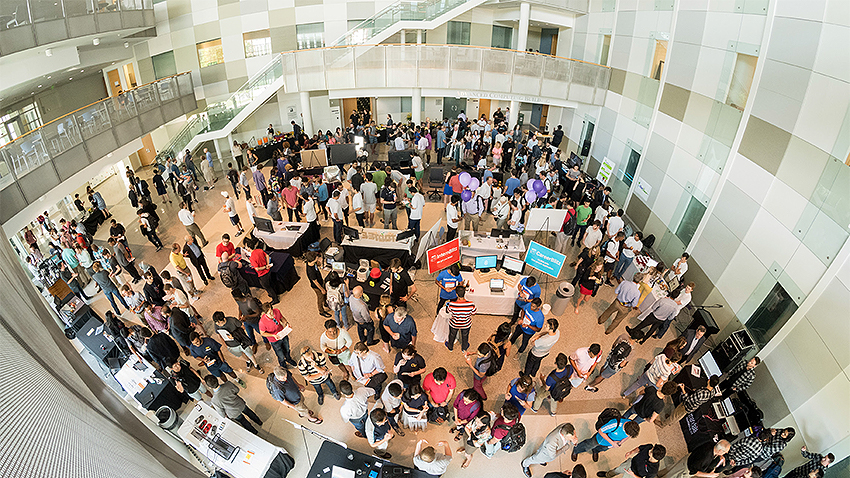
Name: Meenakshi Khosla, Postdoctoral Associate at MIT
Date: Tuesday, March 14, 2023 at 11:00 am
Location: Scheller College of Business, Room 101
Link: This seminar is an in-person event only. However, the seminar will be recorded and uploaded to the School of Computational Science and Engineering channel on Georgia Tech MediaSpace following the presentation.
Title: Towards Data-Driven Modeling in Large-Scale Naturalistic Neuroscience
Abstract: Neuroscience is currently undergoing an explosion in the availability of large-scale brain activity data, so the major challenge no longer lies in data collection, but in deriving understanding from this abundant stream of complex, high-dimensional, noisy data with methods that fully leverage its potential. How can we understand neural representations and infer computational principles from large-scale brain activity data directly? My research group will develop the theory, modeling, and machine learning techniques needed to tackle these challenges, and will provide algorithmically and computationally precise accounts of information processing in the brain. Progress in this research could advance our understanding of biological intelligence and the neural basis of neuropsychiatric disorders, inform novel treatments and neural prostheses, and lead to the development of new approaches to generating machine intelligence.
In this talk, I will present lines of previous and proposed research that highlight the potential of this vision. First, I will present a line of data-driven modeling that revealed the representational structure in the high-level visual cortex and led to the discovery of a neural population selectively responsive to images of food. Second, I will present a modeling framework, called response-optimization, for inferring computations directly from brain activity data with minimal apriori hypotheses. Here, we trained artificial neural network (ANN) models directly to predict the brain activity related to viewing natural images. We then developed techniques for interpreting the networks and characterizing the emergent functional capabilities of these brain response-optimized networks. This work highlights how models trained to capture human brain activity can spontaneously recapitulate human-like behavior. Third, I will present my work on developing neural network models of brain responses across wide-spread cortical regions to dynamic, multi-modal stimuli like movies, with an integrated modeling approach that captured visual attention, multi-sensory auditory-visual interactions and temporal context. Finally, I will propose future directions for leveraging these data-driven computational tools toward i) understanding the representational structure underlying our capacity for high-level cognitive processes like social perception, ii) understanding the granularity of similarity between biological and artificial neural networks, iii) understanding the functional consequences of brain alignment in ANN representations, and iv) understanding not just how the brain is organized or how a process works but fundamentally, why the brain works the way it does.
Bio: Meenakshi Khosla is a postdoctoral associate in the Brain and Cognitive Sciences department at MIT. Her research interests lie at the intersection of neuroscience, artificial intelligence, and large-scale data analysis. Her current projects focus on leveraging large-scale data to develop interpretable machine learning tools for understanding structured neural representations and computations in biological systems. Previously, Meenakshi received her PhD in Electrical and Computer Engineering (ECE) from Cornell University, where she worked broadly at the intersection of machine learning and neuroimaging, developing predictive models to understand the distinctive characteristics of the brains of people affected with different mental disorders.
Event Details
Media Contact
Tasha Thames
tthames6@gatech.edu
EVENTS BY SCHOOL & CENTER
School of Computational Science and Engineering
School of Interactive Computing
School of Cybersecurity and Privacy
Algorithms and Randomness Center (ARC)
Center for 21st Century Universities (C21U)
Center for Deliberate Innovation (CDI)
Center for Experimental Research in Computer Systems (CERCS)
Center for Research into Novel Computing Hierarchies (CRNCH)
Constellations Center for Equity in Computing
Institute for People and Technology (IPAT)
Institute for Robotics and Intelligent Machines (IRIM)